While dealing with data, businesses often need help with the challenge of untangling raw and unstructured information. And, data wrangling skillfully solves this puzzle by being seamlessly laced with ETL processes. This process involves carefully refining and organising data to make sure it is not just ready but also optimal for insightful data analytics by data scientists.
In this article, we will go through the dynamic world of data wrangling, shedding light on its techniques, distinguishing it from ETL processes and uncovering the trends shaping its trajectory in 2024.
Understanding Data Wrangling
Data wrangling, often referred to as data munging or data cleaning, is the unsung hero in data science and analytics. It is the art of refining raw datasets and transforming them into a trove of insights. Imagine gaining access to a treasure chest of new data perspectives, each a potential gem for holistic analysis.
It is the meticulous process of cutting, shaping and polishing these raw data gems, making them ready for transformative insights. In a world of massive, complex datasets, this process turns the unstructured into the interpretable, ensuring your data is not just analyzed but truly understood.
In the process of data wrangling, the artistry lies in actions like combining diverse data sources, bridging gaps and getting rid of stuff that is not required. Sometimes, the data scientists even need to find and fix weird bits in the given data during the process.
A survey found that about 60% of data scientists' effort is spent organizing and cleaning data, and just about 19% is spent gathering data sets. As a result, 80% of their time is spent on data preparation, making it a significant and time-consuming effort. There are various advantages of using automation tools.
Data munging can be done manually or automatically, especially when dealing with huge piles of information. In sizable operations, dedicated data science professionals spearhead the charge, ensuring seamless data refinement. Meanwhile, smaller enterprises lean on non-specialists, underscoring the universal importance of this process.
Necessary Steps to Perform Data Wrangling
Different approaches are required for each data project in order to ensure the availability and reliability of the final dataset. These are often called the necessary data wrangling steps or tasks.
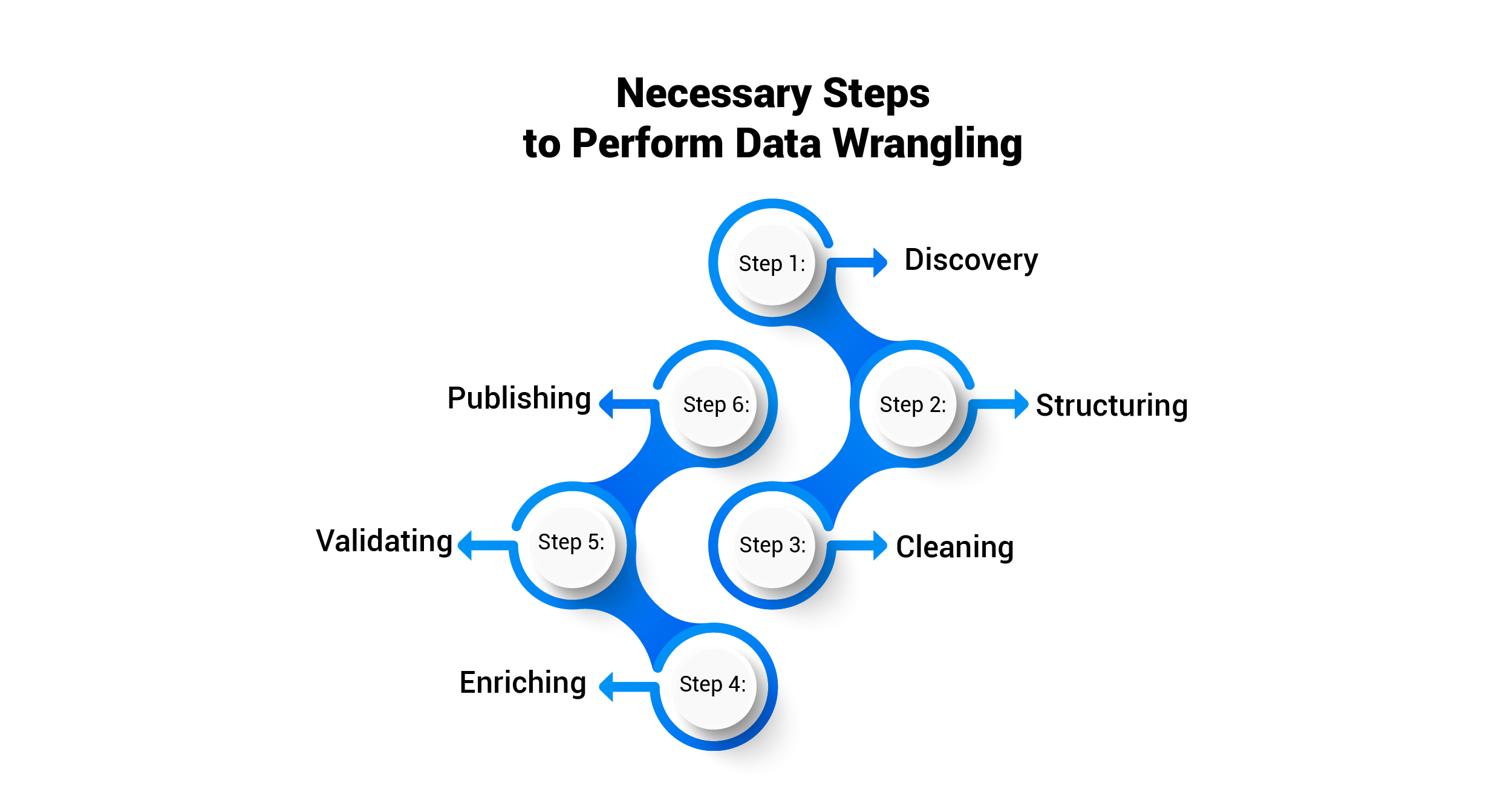
- Step 1: Discovery
One should commence with a comprehensive exploration of the data. Examine its nuances, identifying trends, patterns and potential issues like missing or incomplete values. This initial discovery establishes the groundwork for the next activities. - Step 2: Structuring
The raw and disorganized data must be transformed into a more logical and approachable structure during the structuring process. To improve the data’s usefulness for further analysis, this method involved adding columns, classes or headings. - Step 3: Cleaning
Error correction and outlier removal are the main goals of the cleaning stage, which is essential to maintaining data integrity. It is crucial to ensure that the data is accurate because a cleansed dataset is essential to the success of any further research. - Step 4: Enriching
Enhancing the data adds contextual depth, but it’s optional. To maximize the utility of the cleaned and formatted data for analysis, this strategic procedure includes improving it using techniques like downsampling or upsampling. - Step 5: Validating
The validation step ensures the correctness and consistency of the data. Through meticulous checks, any discrepancies or issues that might impact the accuracy of insights are identified and rectified, certifying the dataset’s readiness for analysis. -
Step 6: Publishing
The publishing phase is the last step in this process. This entails facilitating easy access to the revised dataset, either through seamless integration into pre-existing systems or database integration.
The culmination of these steps guarantees the availability of high-quality data that is ready to support the creation of insightful reports and foster effective communication of findings to stakeholders.
The Importance of Data Wrangling
Data wrangling plays an important role in the dynamic field of data analytics. It takes centre stage in navigating the intricacies of diverse datasets.
- Safeguarding Data Quality
One should commence with a comprehensive exploration of the data. Examine its nuances, identifying trends, patterns and potential issues like missing or incomplete values. This initial discovery establishes the groundwork for the next activities. - Architect of Structure
Consider data munging as the architect shaping raw, disorderly data into a structured, clean and coherent format. It transforms the data into a solid basis that is necessary for insightful and reliable analysis. - Building a Strong Foundation
In the field of data analytics, the absence of a rigorous data wrangling process is akin to constructing a house on an unstable foundation. A dangerous venture that is prone to collapse and yielding unreliable results. -
Streamlining Decision-Making
Data wrangling is essential for combining insights from many data sources, facilitating prompt decision-making and accelerating the generation of valuable insights. It ensures that noisy, flawed, and missing data are meticulously addressed, laying the groundwork for robust data mining.
Data munging is the key to removing complexity and promoting clarity in the complicated world of data analytics. With its deep insights, it provides organizations with the ability to make well-informed decisions and guarantees a solid analytical base.
Data Wrangling vs. ETL
Data management is a dynamic world and distinguishing between data wrangling and ETL (Extract, Transform, Load) is important for an effortless data journey.
Data Wrangling concentrates on preparing unprocessed data for analysis. It thrives on organizing unstructured, disorganized data into flexible, ordered formats. It is an iterative procedure that occurs close to data analysis, adjusting data to suit particular requirements.
On the other hand, ETL directs a thorough data integration ballet. By extracting, processing and loading data, it gives large-scale scenarios automation and structure. For strong business intelligence and synchronized data across systems, ETL is the preferred solution.
Although both guarantee the quality of the data, data wrangling excels in getting the data ready for data analysis, whereas ETL organizes the integration process. When combined, they enable data science experts in data analysis and management to skillfully approach the complex world of analytics.
Data Wrangling Techniques
Efficiency, speed and adaptability are paramount when talking about data wrangling in 2024. A brief look at the methods guiding the direction of the same process in the future is provided by these techniques.
- Automation and AI
Automation is the buzz of modern data wrangling. AI-driven technologies examine data in real time, identifying patterns and outliers, drastically reducing the manual effort in data cleaning. Machine learning algorithms go a step further, suggesting data transformations based on historical patterns. - Data Integration Suppliers
Data munging is made easier by data integration providers’ end-to-end solutions. With their user-friendly interfaces that facilitate complicated data transformations without the need for scripting, these platforms enable data analysis to handle ETL procedures with ease. - Enhancing and Restructuring Data
Data wrangling extends beyond cleaning; it’s about enriching. These days, data science methods enable augmentation with other data sources, providing additional context. Data reshaping facilitates swift pivoting and restructuring to align with specific analysis requirements. -
Real-Time Data Wrangling
Data wrangling is essential for combining insights from many data sources, facilitating prompt decision-making and accelerating the generation of valuable insights. It ensures that noisy, flawed, and missing data are meticulously addressed, laying the groundwork for robust data mining.
The era of real-time data analytics demands this process to be done in real time. Tools capable of processing and cleaning data as it flows in ensure that analytical models are consistently fueled with the latest, high-quality data.
This landscape experiences a paradigm shift in 2024 toward automation, artificial intelligence (AI)-driven methods and user-friendly platforms. These innovations are essential because they address the growing needs of data analytics, particularly in the areas of big data and real-time data analytics.
Trends Shaping Data Wrangling in 2024
Artificial Intelligence (AI) and Machine Learning (ML)
AI and ML act as transformative catalysts, introducing advanced levels of automation and intelligence in the process of data wrangling.
- Advanced AI algorithms automatically filter complex datasets by identifying trends, oddities, and discrepancies which simplifies the process and enhances accuracy.
- ML models recommend data transformations based on historical patterns, reducing manual labour and improving the overall quality of transformations.
- Predictive data enrichment, which is especially useful for unstructured data, is the process by which AI anticipates and fills in missing values in datasets by utilizing external sources.
Big Data Challenges
Big data brings forth a set of challenges and opportunities, influencing how we approach data wrangling.
- Evolving tools ensure scalable procedures to handle the exponential expansion in data, avoiding obstruction.
- The need for quick decision-making drives the development of real-time data cleaning processes, which in turn drives real-time analytics.
- Regardless of the format or source, these technologies have evolved to manage diversity with ease, integrating and converting data.
Data Governance and Compliance
Data governance is critical at a time when data privacy and compliance are given top priority.
- Strict laws mandate careful data processing and handling, with data wrangling integrating measures for compliance.
- Ensuring auditable transformations for data quality and regulatory compliance necessitates thorough tracking. This includes data auditing and provenance.
- Modern data solutions place a strong emphasis on collaboration, making compliance easier through annotation and documentation.
Looking Beyond 2024
Anticipating trends beyond 2024 in the field of data wrangling.
- Additional Automation: As AI and ML algorithms get increasingly complex in handling data issues, automation stays at the forefront.
- Integration with Data Science Platforms: Data wrangling and data science platforms combine seamlessly to create a unified experience for data science professionals.
- Focus on Data Ethics: As privacy laws change, there is a greater focus on data wrangling compliance and ethical issues.
- Data Wrangling as a Specialized Skill: It is becoming more and more important in the data landscape. It emerges as a specialized skill set, with dedicated professionals and certifications.
Conclusion
In summary, data wrangling stands as a linchpin of effective data analytics, effortlessly transforming raw data into actionable insights for informed decision-making. Despite challenges, embracing automation, prioritizing data governance, fostering collaboration and committing to continuous learning are essential strategies to effectively tackle the challenges of this procedure.
Looking forward, data wrangling remains a dynamic field, evolving with demands of big data, artificial intelligence, and ethical considerations. It is a world filled with endless possibilities and those who grasp its intricacies will lead the way in data-driven innovation. The growing importance of this field is evident, ensuring reliable data storage systems and investments in techniques for enhanced user experiences.