In a nascent field like Data science, it can be hard for people to understand what distinguishes a Data Scientist from a Data Analyst. Data Science, one of the most glamourized profession right now, has seen a recent boom for its wonderful insights about customer, product & marketing. Although many of the responsibilities of analysts & scientists closely match, but there are major differences to recognize.
Data scientists understands the data from a business perspective & makes predictions for accurate decisions. Data analysts are responsible for various tasks such as to collect, organize data & obtain statistical information from them. They perform the presentation of the Data & builds reliable Databases for different companies.
Both Data analysts & scientists focuses on the market & understands the hidden business trends to forecast the future of the business. Both have a crucial place in business, one cannot be successful without the presence of the other.
Unfortunately, there is no industry standard usage of the terms “Data Analyst” and “Data Scientist” that clearly distinguishes between the two roles. However, the devil is in the details; these roles tend to be complementary to one another but often span a wide variety of different skill sets and functional roles. For instance, within the conceptual data mining lifecycle illustrated in Figure 1 below, a “Data Analyst” focuses on the movement and interpretation of data, typically with a focus on the past and present. Alternatively, a “Data Scientist” may be primarily responsible for summarizing data in such a way as to provide forecasting, or an insight into future based on the patterns identified from past and current data.
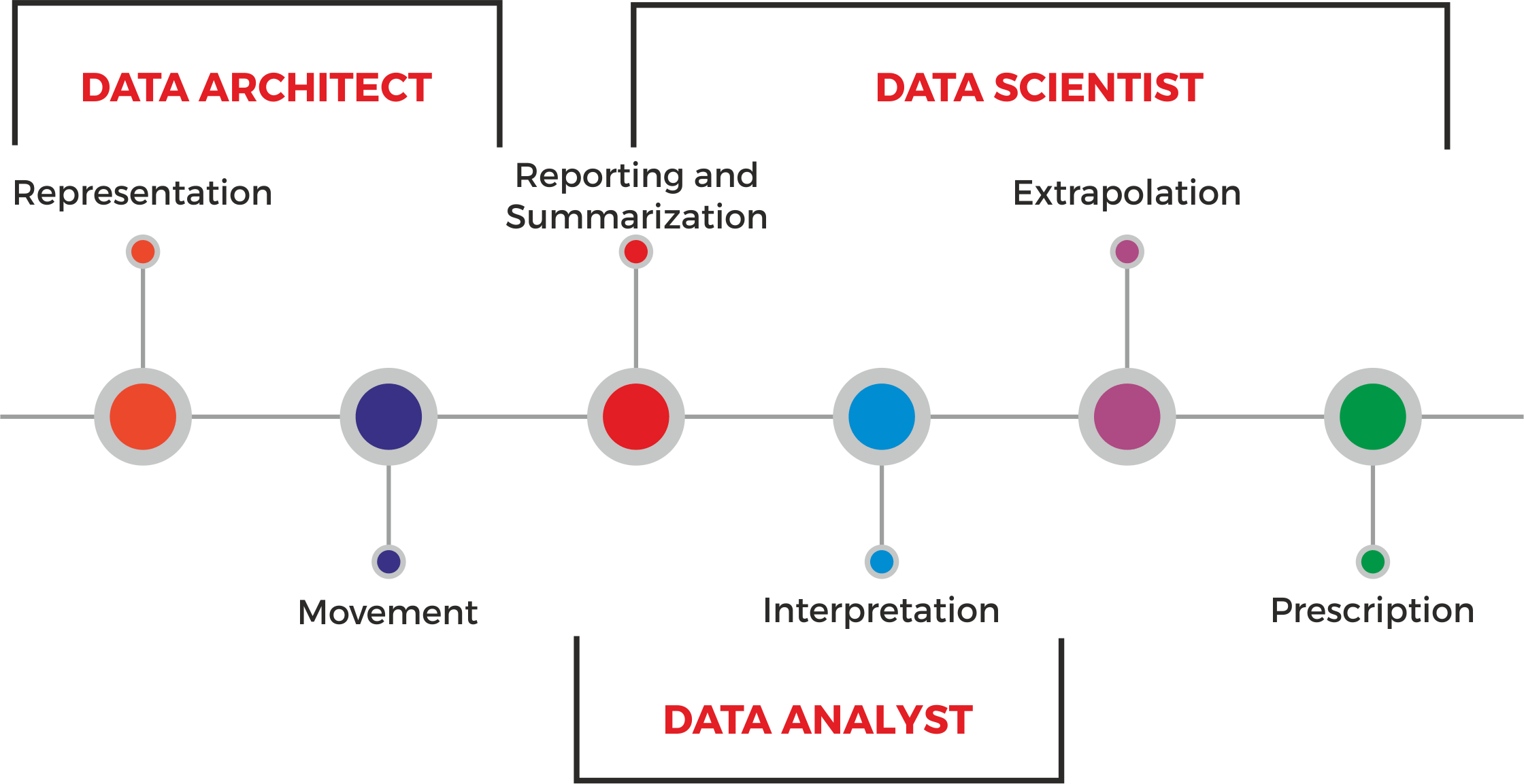
Data Analyst | Data Scientist |
---|---|
How do I solve reporting performance problems? | How do I balance model robustness with the simplicity of the message? |
How do I solve data quality and sourcing problems? | How do I detect and quantify changing relationships? |
What tools and reports are contextually appropriate? | How can I correct for biased and incomplete data? |
How will my analysis be used by my clients? | How do I effectively communicate model uncertainty? |
How can I learn more about my client's business? | How do I help my client trust the model? |
Data Analyst are... | Data Scientist are... |
---|---|
BI Developers | Data Mining Experts |
SQL Developers | Statistics SMEs |
Visual Analytics Users | Trusted Advisors |
Data Mining Tool Users | Experiment Designers |
Report Owners | Advanced Analytics Software Experts |
Data Analyst- Focus on describing the past | Data Scientist- Focus on improving the future |
---|---|
I have found a way to increase the speed of reporting an average of 70% by tuning large tables and indexes. | Our model quantifies cyclical patters in call volume. We can save $220k a year by aligning staffing to these cycles. |
I have noticed that there is more missing and incomplete device log data from customers in rural areas. | A CRM data overlay will improve IVR routing in a way that increases customer acceptance and saves $250k per year. |
Are the reports we are sending you useful? Here are some ideas for new reports that are easier to view and understand. | I can predict 82% +/- 5% of future call volume 1 month in advance with 90% confidence using currently accessible data. I believe that I can increase this to 86% +/- 4% with a model enhancement. |
Source Chapman, Pete, et al. "CRISP-DM 1.0 Step-by-step data mining guide."(2000).
http://www.sv-europe.com/crisp-dm-methodology/