The business world’s unquenchable thirst for data has led to the need to efficiently harvest and process it to deliver actionable business outcomes. This in itself, has become a rapidly growing micro industry, crawling and scraping the web for data to outperform the competition and drive sustainable growth. Vendors have blossomed, not unlike mushrooms in the wild, to provide more data. It’s madness out there, but as Data Scientists, we’re expected to make sense of the seemingly senseless.
Enter the world of integrated Data Infrastructure, where all relevant data points and datasets are seamlessly ingested and processed, available on demand and in real time, in easily consumable form, to business functions and employees to get ahead in the ever disruptive and constantly evolving corporate landscape, worldwide.
From a concept point of view, it’s not very different from standard ICT infrastructure models, but the nuances are vast in number and complexity. Here’s a closer look at what comprises an enterprise data infrastructure, what its functionalities are, and how the whole can deliver greater value than the sum of its parts.
Architecture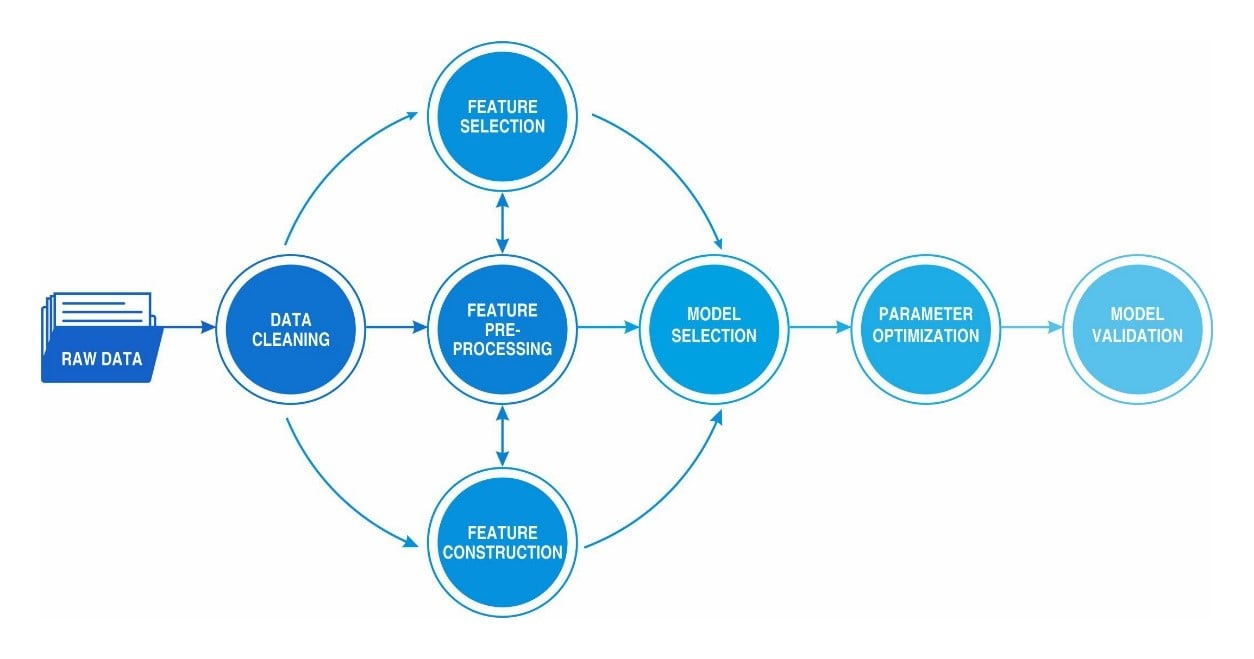
In the above diagrammatic representation, raw data passes through the cleansing or munging phase, preparing it for three different segments, the primary one being data feature preprocessing, alongside parallel outputs of feature selection and feature construction, as per the exact needs of the business. This paves the way for the data model selection, which is what will be the platform for processing future data. Parameter optimization and normalization, alongside identifying outliers form the next step in the architecture, with the final data model validation being the penultimate outcome.
Note: This is a single simple example scenario and there may be more infinitely complicated ones depending on the complexities of the use cases in question.
As with any structure, the architecture is the first and primary process to validate according to business needs. Does the enterprise gather fixed datasets? Does it store in warehouses or data lakes? How does it handle streaming data analyses? And most importantly, how does it break silos to get the data derived insights right into where they want them to be?
Answers: The cross platform and interconnectedness of data processing forms the core of the architecture, especially when you’re looking at an analytics and neural network driven experimental architecture (EDA model) explained in the diagram below:
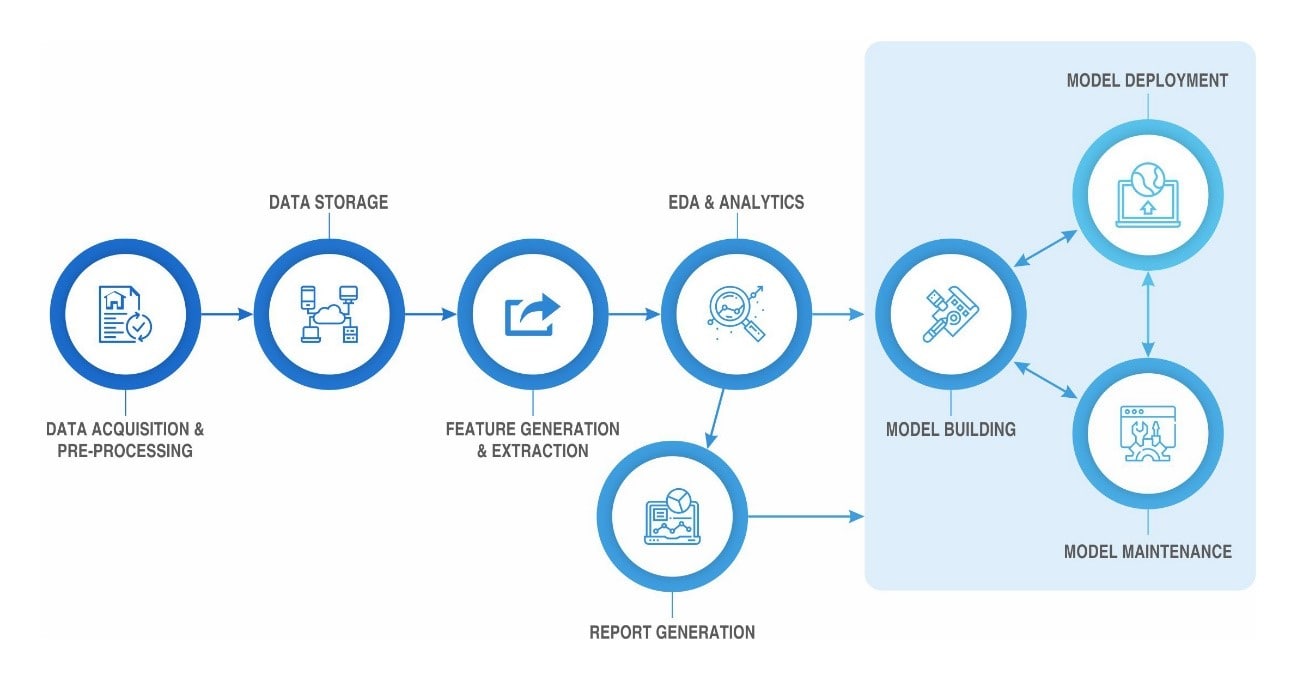
With a clear understanding of the architecture, let’s move into the actual structural components that will shape the data infrastructure in your organization:
Structural ComponentsAs with any infrastructure, the desired outcome determines the design. Though most enterprises kick off their data infrastructure with on-premise servers, there are several among them that prefer a cloud deployment, and the rate of adoption for this is increasing rapidly.
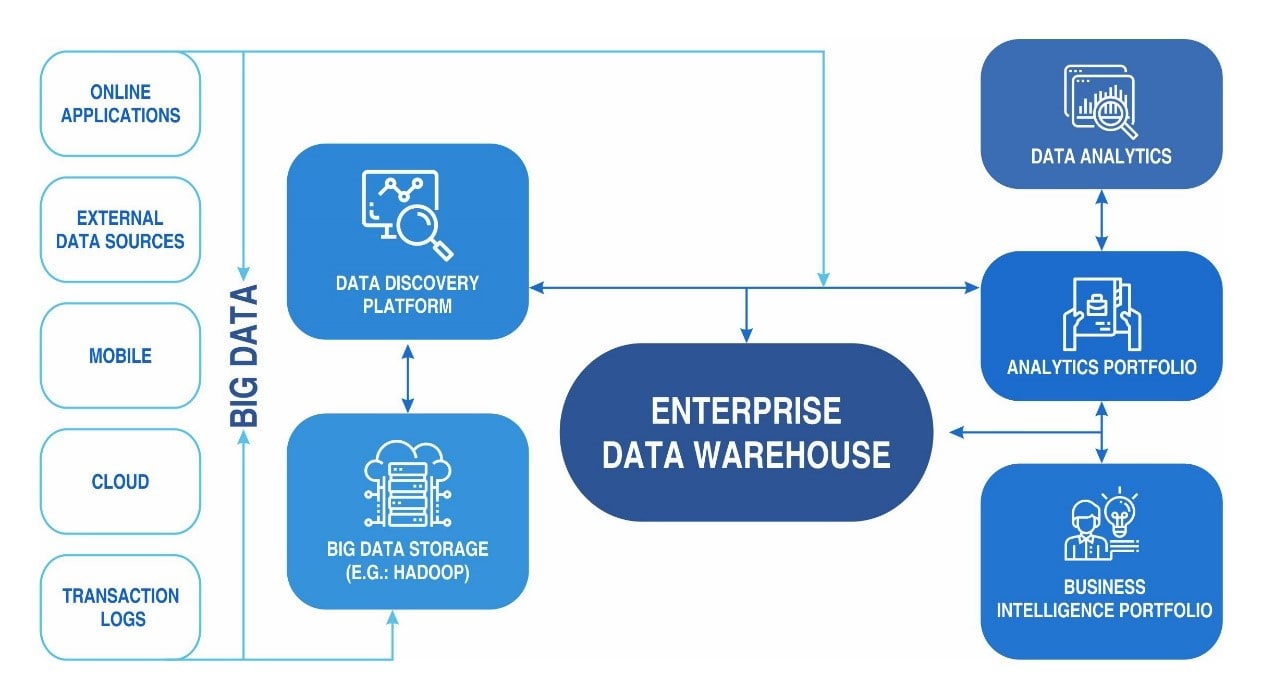
The key components to consider while building a data infrastructure ought to be validated from both ends of the spectrum, the business requirement and the data models currently in use. However, from a high level overview, the structure can be classified as:
Discovery - This component refers to the acquisition of data. This could be from a large variety of sources including online applications, mobile applications, transactional data, acquired data and other types of user data, both online and offline. This usually feeds into the next component- the storage.
Storage - Storing data may have become cost effective in recent times, but it is also infinitely more complex. While small organizations rely heavily on relational databases, scaling up storage to accommodate growing business needs may at times warrant a data lake, or a large enterprise grade database that provides warehousing capabilities. Most large organizations follow the ETL model of extraction, transformation and loading of the data.
OLAP- With the advent of cloud computing, online, real-time analytical processing of transactional data has become the norm, over the exception. This component in the data architecture processes the data and provides the necessary preparation for the final stage in the process - the consumption of data.
Visualization/Consumption - This is where it all bears fruit. Largely comprising business intelligence and data visualization tools, the data is presented to stakeholders for analysis and insight gathering, and in most cases in large companies, for further processing with advanced AI and Data Science tools. In most cases, clusters move on to several cleansing and munging properties, which are then incorporated into the existing development tools, checked for persistence in accuracy and then finally produced (production level at employee or management).
Data Infrastructure maps, of which the aforementioned is just a symbolic representation, can run into hundreds of more complicated data models, based on the complexities of the data model determined at the architectural stage.
For all of the above, knowledge, combined with a viable professional credential is key. If this does not happen, it would be like dreaming data all day and the limitless possibilities to improve business scenarios without really having any clue how to go about it. This could be the perfect reason to get on board the world’s largest and most advanced data science certification authority and get knowledge, skills and concepts validated by the data science authority that corporate leaders across the world trust. Check out further details before it’s too late!