The era of intuitive business decisions is officially over. As humans, we frequently rely on intuition and emotion when making quick decisions, but today's landscape demands a much smarter, data-centric approach. Organizations, irrespective of their size or industry, need to utilize data for informed decision-making. Enter data-driven decision-making!
This strategy of decision-making harnesses metrics and insights derived from data to guide critical business decisions in alignment with your organizational goals and strategies.
So, how to use it to make informed decisions? Continue reading to explore the concept of data-driven decision-making is, its benefits, and the essential steps to enable it.
What is Data-Driven Decision Making?
Data-driven decision making can be defined as a methodical approach to problem-solving where actions are guided by insights extracted from data analytics solutions. Implementing data-driven decisions calls for the establishment of various systems, including those for data collection, data processing, data visualization, and more.
Data-driven decision making involves defining and tracking Key Performance Indicators (KPIs) in areas such as business impact, customer perspective, and project success. This includes metrics like revenue growth rate (business KPIs), addressing customer complaints (customer KPIs), and measuring project outcomes (project output KPIs).
Types of Decision Making
There are different types of decisions you can take to leverage data-driven decision-making for your organization:
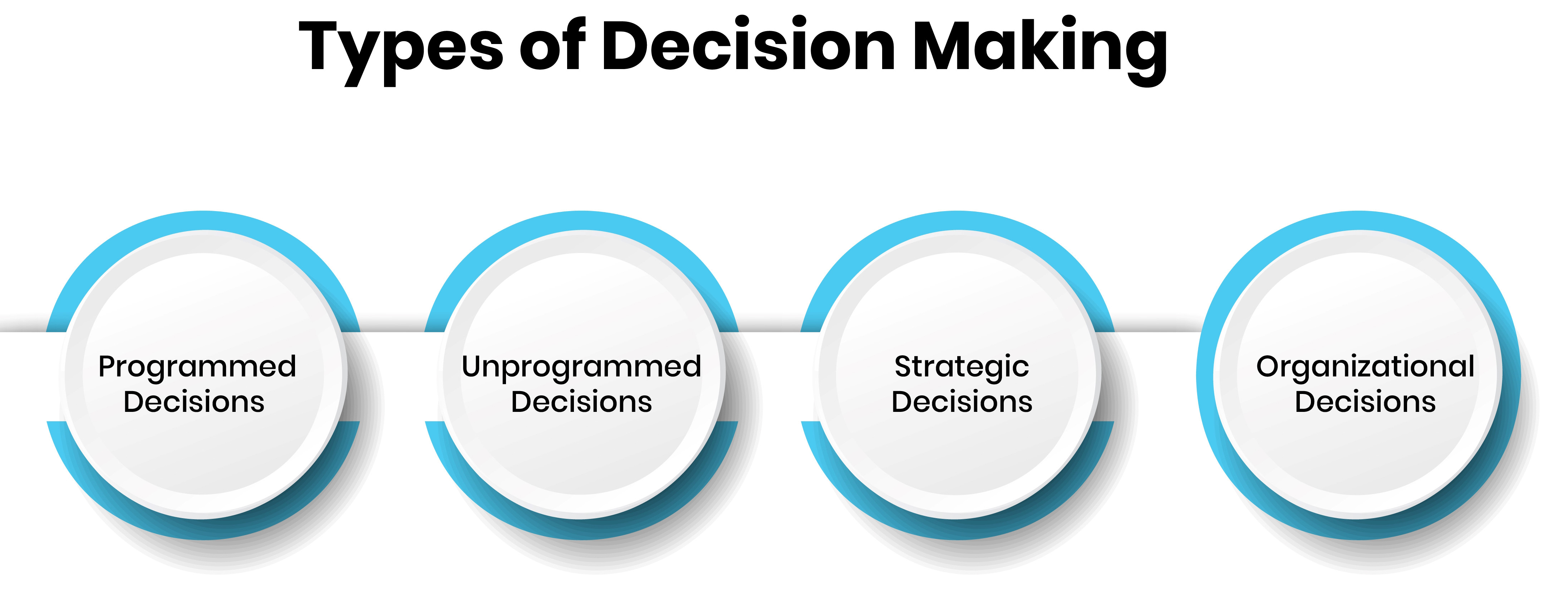
-
01. Programmed Decisions:
- Based on past data or experience to achieve well-defined outcomes.
- Long-term decisions forming part of business strategy.
- Utilize descriptive and diagnostic analytics to measure impact.
-
02. Unprogrammed Decisions:
- Short-term decisions requiring data analysis.
- Tactical or operational decisions.
- Leverage prescriptive and predictive analytics.
-
03. Strategic Decisions:
- Long-term planning decisions.
- Shape the organization's direction and mission.
-
04. Organizational Decisions:
- Data-driven decisions requiring data analysis.
- Used by multiple individuals in the organization.
Each type of decision-making involves utilizing data analytics tools and methodologies to inform and drive decision-making processes effectively.
How Data-Driven Decision Making Works?
Data-driven decision-making operates by providing clarity to employees, aiding them in comprehending the problem at hand and pinpointing specific areas requiring attention. This process begins with the collection and categorization of pertinent data, streamlining the effort and time expended.
Subsequently, the data is systematically organized, processed, and analyzed utilizing statistical techniques, machine learning algorithms, and visualization tools. Decision-makers, by evaluating both qualitative and quantitative factors, can discern objectives and devise incremental strategies to attain them. The central emphasis lies in selecting and extracting insights from relevant data sources to inform and bolster decision-making endeavors.
Why Data-Driven Decision Making is Important?
A survey conducted by Drexel University’s LeBow College of Business revealed that 77 percent of professionals specializing in data and analytics consider prioritizing data-driven decision-making as a key objective within their data programs. Benefits of data-driven decision-making include:
-
Analytical Problem Solving
Enables organizations to adopt an analytical approach to problem-solving, fostering a value-centric perspective through actionable insights. -
Digital Transformation
Decisions based on data facilitate the adoption of a digital approach, allowing stakeholders to make well-informed decisions promptly, leading to a competitive advantage in terms of speed, agility, and scale. The Statistica report forecasts that by 2026, global spending on digital transformation will reach USD 3.4 trillion. -
Faster, Better, Informed Decision
When human intuition integrates with data, it results in superior business decisions, reducing bias introduced by gut feelings and improving overall outcomes. -
Efficient Resource Utilization
With data-driven decision making, you can save time and resources by minimizing human input and associated risks, leading to more efficient decision-making processes. -
Objective Decision Making
Data-driven decisions remove bias and subjectivity as it relys solely on factual analysis. -
Increased Data Visibility
Enhances data transparency by making data available to multiple stakeholders, promoting easy sharing of information. -
Enhanced Data Literacy
Supports data professionals in improving their data literacy skills, opening better career opportunities in the evolving data-driven landscape. -
Increased Customer Satisfaction
Gathering multiple data points about customers enables companies to devise strategies for enhancing overall satisfaction, leading to improved retention rates and future revenue growth. A significant 74 percent of CX leaders emphasize the importance of enhancing content and knowledge delivery to both customers and employees. -
Increased Data Accessibility
It establishes data access for a broader audience, reducing the likelihood of errors due to a lack of information and encouraging data sharing. -
Competitive Advantage
Leveraging data effectively provides insights into the market, enabling informed decisions and a competitive edge. Companies that embrace data-driven approaches are significantly more likely to outperform their competitors, being 23 times more likely to excel in customer acquisition, approximately 19 times more likely to maintain profitability, and nearly seven times more likely to retain customers.
Examples of Major Brands Using Data
Coca-Cola uses data analytics to enhance its customer experience across various facets of its operations, including marketing campaigns, content creation, product development, and sales strategies. By leveraging data insights, the company can tailor its offerings to meet customer preferences effectively, thereby fostering loyalty and satisfaction among its consumer bases.
Google utilizes data-driven insights to optimize internal performance, particularly in identifying behaviors correlated with effective managerial performance. This approach enables Google's senior leadership to support managers in enhancing their engagement, performance, and longevity within the company, ultimately reducing talent acquisition costs while maintaining a high-performing workforce.
Lufthansa, the world's second-largest airline by passenger volume, improved its analytics coherence. Utilizing a dedicated analytics platform led to a substantial 30% revenue boost companywide. This surge resulted from precise data collection, analysis, and subsequent data-driven decisions by employees.
Amazon exemplifies the transformative impact of data-driven decision-making on business success. By prioritizing data-driven operations, Amazon has disrupted traditional retail paradigms, achieving remarkable growth and a market capitalization of USD 1 trillion. Data serves as the cornerstone for strategic decision-making, guiding every significant project approval and operational goal, and solidifying Amazon's position as a leader in the global marketplace.
Steps To Enable Data-Driven Decision Making
To enable effective data-driven decision-making within an organization, you need to follow a structured approach encompassing five key steps that go as:
- Step 1: Involves defining the problem comprehensively, aligning business goals, and understanding the contextual landscape. Collaborative efforts between data teams and business users ensure that proposed solutions directly address business needs.
- Step 2: Revolves around collecting relevant data from diverse internal and external sources. This entails leveraging existing data repositories and exploring additional datasets to uncover meaningful insights beyond conventional sources.
- Step 3: Focuses on analyzing the collected data to identify patterns, anomalies, and correlations. Empowering business users to engage directly with data facilitates early identification of potential gaps and enhances adoption and value realization.
- Step 4: Entails developing and implementing a strategic plan to address the identified problem. This involves setting measurable goals, defining appropriate KPIs, and establishing a timeline for execution. Involving business stakeholders from the outset ensures alignment with organizational objectives.
- Step 5: Involves evaluating the outcomes of the implemented plan by tracking key performance indicators. This iterative process not only assesses the effectiveness of the solution in addressing the initial challenge but also provides insights for future enhancements and informs more informed decision-making.
Conclusion
Data accuracy and relevance are paramount for informed decisions, guarding against errors and inefficiencies thus it is important to cultivating a culture of critical thinking that empowers employees to extract meaningful insights from data, enhancing decision-making processes.